The Power of Data in AI: Unlocking the Potential for Smarter Systems
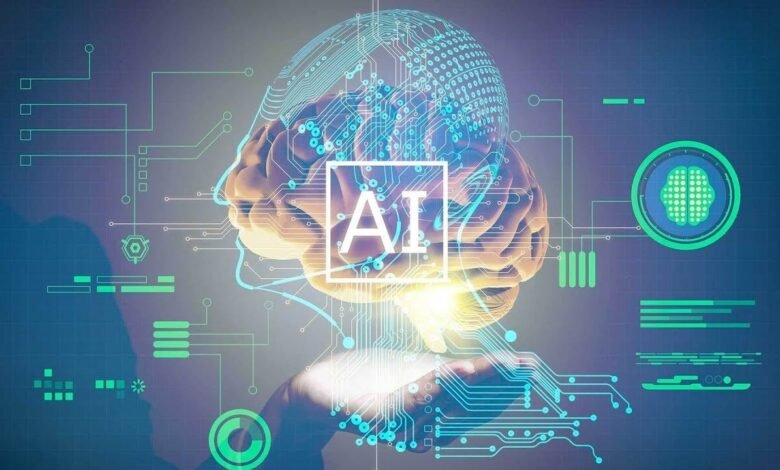
The power of data in AI is unparalleled, serving as the bedrock upon which the intelligence of systems is built. In today’s digital age, data fuels the advancements in artificial intelligence, unlocking the potential for smarter, more adaptive systems. From training algorithms to making real-time decisions, data plays a pivotal role in shaping the capabilities of AI technologies. With the exponential growth of data across various domains, harnessing its potential has become essential for driving innovation and achieving breakthroughs in AI development.
As organizations increasingly rely on AI to automate processes, analyze vast amounts of information, and improve decision-making, the significance of data in AI cannot be overstated. It forms the foundation upon which AI algorithms learn, adapt, and evolve, enabling them to perform complex tasks with precision and efficiency. By understanding the transformative power of data in AI, we can harness its potential to revolutionize industries, drive economic growth, and address some of society’s most pressing challenges.
The Power of Data in AI
At its core, AI relies on data to learn, adapt, and make informed decisions. There are three primary types of data used in AI development: training data, testing data, and validation data. Training data is used to teach AI algorithms, testing data evaluates the model’s performance, and validation data ensures the model’s accuracy and generalization.
Quality Data: A Key Component
The Power of Data in AI: The quality of data is paramount in AI development. Without quality data, AI algorithms may produce inaccurate or biased results. It is essential to ensure data accuracy, completeness, and integrity throughout the data lifecycle to build reliable AI systems.
Data Collection Methods
The Power of Data in AI: Data can be collected through various methods, including manual collection, automated collection, and crowdsourcing. Each method has its advantages and challenges, and the choice depends on the specific requirements of the AI project.
Data Preprocessing
The Power of Data in AI: Before feeding data into AI algorithms, it undergoes preprocessing to clean, format, and normalize it. Data preprocessing also involves handling missing data and outliers to improve the quality of the dataset.
Data Labeling and Annotation
The Power of Data in AI: Data labeling is the process of annotating data with labels or tags to provide context for AI algorithms. Techniques such as supervised learning rely heavily on accurately labeled data to train models effectively.
Data Augmentation
The Power of Data in AI: Data augmentation involves creating additional training data from existing datasets by applying transformations or perturbations. This technique helps improve model performance and generalization by exposing the model to a diverse range of data.
Data Storage and Management
The Power of Data in AI: Choosing the right storage solutions is crucial for efficiently managing large volumes of data in AI projects. Additionally, data security and privacy considerations must be addressed to protect sensitive information from unauthorized access or misuse.
Data Governance and Compliance
The Power of Data in AI: Data governance frameworks ensure that data is managed responsibly and ethically. Compliance with regulatory requirements, such as GDPR and CCPA, is essential to mitigate risks associated with data misuse and ensure transparency in data handling practices.
Data Analysis and Interpretation
The Power of Data in AI: Data analysis involves extracting meaningful insights and patterns from large datasets to inform decision-making. Advanced analytics tools, such as machine learning and data visualization techniques, facilitate the analysis and interpretation of complex data Types.
The Impact of Big Data on AI
The Power of Data in AI: The proliferation of big data has revolutionized AI by providing access to vast amounts of information for training and testing purposes. However, leveraging big data also presents challenges related to data storage, processing, and privacy.
Data-driven Decision Making
The Power of Data in AI: AI utilizes data to make informed decisions across various domains, including healthcare, finance, and marketing. By analyzing data in real-time, AI systems can automate processes, optimize workflows, and improve overall efficiency.
Ethical Considerations in Data Usage
The Power of Data in AI: Ethical considerations are paramount in AI development to ensure fairness, transparency, and accountability. Addressing bias and discrimination in AI algorithms and promoting diversity and inclusion are essential for building ethical AI systems.
Future Trends in Data-driven AI
The Power of Data in AI: The future of AI is closely intertwined with advancements in data processing technologies, such as edge computing, federated learning, and quantum computing. These innovations will drive the evolution of data-driven AI systems, unlocking new possibilities and applications.
Read More: Facebook is using AI to supercharge the algorithm
Conclusion
The symbiotic relationship between data and AI is fundamental to the advancement of technology and the creation of smarter systems. As we delve deeper into the digital era, the sheer volume and diversity of data available offer unprecedented opportunities for innovation and progress. By leveraging the power of data-driven AI, we can tackle complex challenges, optimize processes, and enhance decision-making across industries.
Looking ahead, the future of AI holds boundless possibilities, fueled by ongoing advancements in data processing technologies and machine learning algorithms. As we continue to unlock the potential of data in AI, it is imperative to prioritize ethical considerations, address biases, and ensure transparency in AI development. By harnessing the transformative power of data responsibly, we can shape a future where AI-driven systems empower individuals, businesses, and societies to thrive in an increasingly interconnected world.